Senior Research Associate
Research
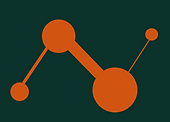

Network meta-analysis
My initial research in the field of network meta-analysis involved using ideas from the theory of complex networks to analyse the affect of network geometry on the performance of Bayesian NMA. I employed computer simulations to generate synthetic trial data and perform Markov Chain Monte Carlo (MCMC) methods (details of this code can be found on my GitHub page). The results of these simulations exposed how bias can enter into evaluation of treatments in an NMA and were used to suggest a method for planning future trials which will best compliment an existing network of evidence. For details of this project, see our publication.
​
I am also interested in introducing NMA to the statistical physics community and exploring how these disciplines can benefit from shared knowledge and ideas. In a recent project we introduce a novel analogy between NMA and random walks. A random walk on a network is a stochastic process consisting of a series of hops between nodes that are connected by an edge. We define a random walk on the network of evidence and use this analogy to overcome the limitations of an algorithm used in NMA software. This project was performed in collaboration with researchers at the University of Freiburg. Details can be found in our publication.
I hope to develop and explore this analogy in the future in order to gain further insight into NMA methodology.
​
Survival analysis
I have also been working on a survival analysis project in collaboration with Prof Anthony CC Coolen at Radbound University in The Netherlands. Survival analysis is a field of medical statistics that involves modelling the probability of survival until some specified event of interest. Of particular clinical interest is the prediction of patient-specific survival probabilities based on a set of observed biomarkers or 'covariates'. In this work, we develop a novel approach to survival prediction based on covariates that are measured over time until some subject-specific final observation time before the event of interest. These predictions are known as 'dynamic' since they can be updated as more observations become available. Our approach aims to overcome some of the limitations of standard dynamic prediction methods. For details of this project, see our recent pre-print.
Software
As of version 2.0-0 I am co-author of the frequentist NMA R-package netmeta. See here for the announcement of version 2.0-0 detailing the main changes.
Other topics of interest
Completing a masters project in collaboration with the School of Health Sciences (UoM) fuelled my desire to work in an interdisciplinary field. While I particularly enjoy the work involved in theoretical physics, mathematics and computing, I am motivated by projects that have direct real world applications. My masters project introduced me to medical statistics and the array of interesting problems that it presents due to the high complexity and dimensionality of clinical data.
My PhD work has focussed on the fields of network meta-analysis and survival analysis, but other topics that have sparked my interest include causal inference, multi-state models and epidemic modelling. In addition, I think the communication of statistics, especially relating to medical data, is extremely important, both within the scientific community and to the general public. I am therefore interested in how to communicate results to different groups in a clear and reliable way.
​
I am also interested in gender bias in medical research and data. While significant improvements have been made in recent years, there are still issues involving gender disparity in study populations, funding of women's health research and reporting of gender-related data. I am interested in using medical statistics to analyse and highlight such problems with an aim to address medical inequalities.
MPhys Project
My masters project, 'Electrical Impedence of the Breast', was completed in collaboration with the Division of Informatics, Imaging and Data Sciences in the School of Health Sciences at the University of Manchester.